Defending SOCs Under Siege: Battling Adversarial AI Attacks
Join our daily and weekly newsletters for the latest updates and exclusive content on industry-leading AI coverage. Learn More
With 77% of enterprises already victimized by adversarial AI attacks and eCrime actors achieving a record breakout time of just 2 minutes and 7 seconds, the question isn’t if your Security Operations Center (SOC) will be targeted — it’s when.
As cloud intrusions soared by 75% in the past year, and two in five enterprises suffered AI-related security breaches, every SOC leader needs to confront a brutal truth: Your defenses must either evolve as fast as the attackers’ tradecraft or risk being overrun by relentless, resourceful adversaries who pivot in seconds to succeed with a breach.
Combining generative AI (gen AI), social engineering, interactive intrusion campaigns and an all-out assault on cloud vulnerabilities and identities, attackers are executing a playbook that seeks to capitalize on every SOC weakness they can find. CrowdStrike’s 2024 Global Threat Report finds that nation-state attackers are taking identity-based and social engineering attacks to a new level of intensity. Nation-states have long used machine learning to craft phishing and social engineering campaigns. Now, the focus is on pirating authentication tools and systems including API keys and one-time passwords (OTPs).
“What we’re seeing is that the threat actors have really been focused on…taking a legitimate identity. Logging in as a legitimate user. And then laying low, staying under the radar by living off the land by using legitimate tools,” Adam Meyers, senior vice president counter adversary operations at CrowdStrike, told VentureBeat during a recent briefing.
Cybercrime gangs and nation-state cyberwar teams continue sharpening their tradecraft to launch AI-based attacks aimed at undermining the foundation of identity and access management (IAM) trust. By exploiting fake identities generated through deepfake voice, image and video data, these attacks aim to breach IAM systems and create chaos in a targeted organization.
The Gartner figure below shows why SOC teams need to be prepared now for adversarial AI attacks, which most often take the form of fake identity attacks.
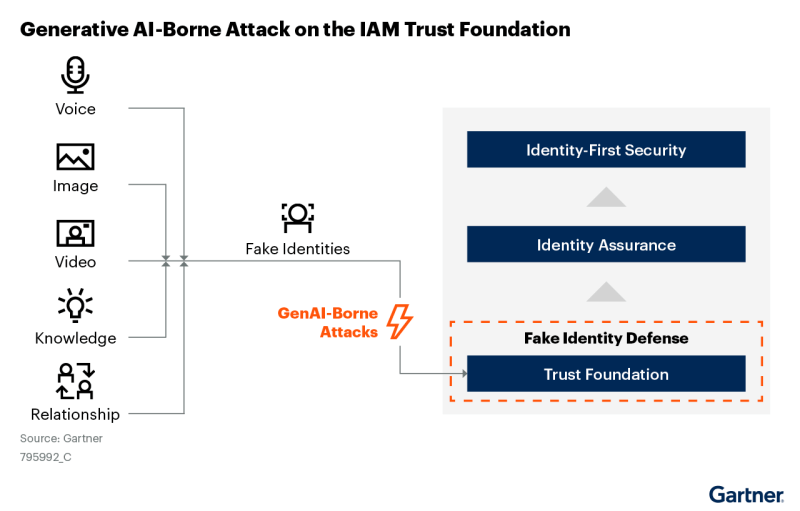
Source: Gartner 2025 Planning Guide for Identity and Access Management. Published on October 14, 2024. Document ID: G00815708.
Scoping the adversarial AI threat landscape going into 2025
“As gen AI continues to evolve, so must the understanding of its implications for cybersecurity,” Bob Grazioli, CIO and senior vice president of Ivanti, recently told VentureBeat.
“Undoubtedly, gen AI equips cybersecurity professionals with powerful tools, but it also provides attackers with advanced capabilities. To counter this, new strategies are needed to prevent malicious AI from becoming a dominant threat. This report helps equip organizations with the insights needed to stay ahead of advanced threats and safeguard their digital assets effectively,” Grazioli said.
A recent Gartner survey revealed that 73% of enterprises have hundreds or thousands of AI models deployed, while 41% reported AI-related security incidents. According to HiddenLayer, seven in 10 companies have experienced AI-related breaches, with 60% linked to insider threats and 27% involving external attacks targeting AI infrastructure.
Nir Zuk, CTO of Palo Alto Networks, framed it starkly in an interview with VentureBeat earlier this year: Machine learning assumes adversaries are already inside, and this demands real-time responsiveness to stealthy attacks.
Researchers at Carnegie Mellon University recently published “Current State of LLM Risks and AI Guardrails,” a paper that explains the vulnerabilities of large language models (LLMs) in critical applications. It highlights risks such as bias, data poisoning and non-reproducibility. With security leaders and SOC teams increasingly collaborating on new model safety measures, the guidelines advocated by these researchers need to be part of SOC teams’ training and ongoing development. These guidelines include deploying layered protection models that integrate retrieval-augmented generation (RAG) and situational awareness tools to counter adversarial exploitation.
SOC teams also carry the support burden for new gen AI applications, including the rapidly growing use of agentic AI. Researchers from the University of California, Davis recently published “Security of AI Agents,” a study examining the security challenges SOC teams face as AI agents execute real-world tasks. Threats including data integrity breaches and model pollution, where adversarial inputs may compromise the agent’s decisions and actions, are deconstructed and analyzed. To counter these risks, the researchers propose defenses such as having SOC teams initiate and manage sandboxing — limiting the agent’s operational scope — and encrypted workflows that protect sensitive interactions, creating a controlled environment to contain potential exploits.
Why SOCs are targets of adversarial AI
Dealing with alert fatigue, turnover of key staff, incomplete and inconsistent data on threats, and systems designed to protect perimeters and not identities, SOC teams are at a disadvantage against attackers’ growing AI arsenals.
SOC leaders in financial services, insurance and manufacturing tell VentureBeat, under the condition of anonymity, that their companies are under siege, with a high number of high-risk alerts coming in every day.
The techniques below focus on ways AI models can be compromised such that, once breached, they provide sensitive data and can be used to pivot to other systems and assets within the enterprise. Attackers’ tactics focus on establishing a foothold that leads to deeper network penetration.
- Data Poisoning: Attackers introduce malicious data into a model’s training set to degrade performance or control predictions. According to a Gartner report from 2023, nearly 30% of AI-enabled organizations, particularly those in finance and healthcare, have experienced such attacks. Backdoor attacks embed specific triggers in training data, causing models to behave incorrectly when these triggers appear in real-world inputs. A 2023 MIT study highlights the growing risk of such attacks as AI adoption grows, making defense strategies such as adversarial training increasingly important.
- Evasion Attacks: These attacks alter input data in order to mispredict. Slight image distortions can confuse models into misclassifying objects. A popular evasion method, the Fast Gradient Sign Method (FGSM), uses adversarial noise to trick models. Evasion attacks in the autonomous vehicle industry have caused safety concerns, with altered stop signs misinterpreted as yield signs. A 2019 study found that a small sticker on a stop sign misled a self-driving car into thinking it was a speed limit sign. Tencent’s Keen Security Lab used road stickers to trick a Tesla Model S’s autopilot system. These stickers steered the car into the wrong lane, showing how small, carefully crafted input changes can be dangerous. Adversarial attacks on critical systems like autonomous vehicles are real-world threats.
- Exploiting API vulnerabilities: Model-stealing and other adversarial attacks are highly effective against public APIs and are essential for obtaining AI model outputs. Many businesses are susceptible to exploitation because they lack strong API security, as was mentioned at BlackHat 2022. Vendors, including Checkmarx and Traceable AI, are automating API discovery and ending malicious bots to mitigate these risks. API security must be strengthened to preserve the integrity of AI models and safeguard sensitive data.
- Model Integrity and Adversarial Training: Without adversarial training, machine learning models can be manipulated. However, researchers say that while adversarial training improves robustness it requires longer training times and may trade accuracy for resilience. Although flawed, it is an essential defense against adversarial attacks. Researchers have also found that poor machine identity management in hybrid cloud environments increases the risk of adversarial attacks on machine learning models.
- Model Inversion: This type of attack allows adversaries to infer sensitive data from a model’s outputs, posing significant risks when trained on confidential data like health or financial records. Hackers query the model and use the responses to reverse-engineer training data. In 2023, Gartner warned, “The misuse of model inversion can lead to significant privacy violations, especially in healthcare and financial sectors, where adversaries can extract patient or customer information from AI systems.”
- Model Stealing: Repeated API queries can be used to replicate model functionality. These queries help the attacker create a surrogate model that behaves like the original. AI Security states, “AI models are often targeted through API queries to reverse-engineer their functionality, posing significant risks to proprietary systems, especially in sectors like finance, healthcare and autonomous vehicles.” These attacks are increasing as AI is used more, raising concerns about IP and trade secrets in AI models.
Reinforcing SOC defenses through AI model hardening and supply chain security
SOC teams need to think holistically about how a seemingly isolated breach of AL/ML models could quickly escalate into an enterprise-wide cyberattack. SOC leaders need to take the initiative and identify which security and risk management frameworks are the most complementary to their company’s business model. Great starting points are the NIST AI Risk Management Framework and the NIST AI Risk Management Framework and Playbook.
VentureBeat is seeing that the following steps are delivering results by reinforcing defenses while also enhancing model reliability — two critical steps to securing a company’s infrastructure against adversarial AI attacks:
Commit to continually hardening model architectures. Deploy gatekeeper layers to filter out malicious prompts and tie models to verified data sources. Address potential weak points at the pretraining stage so your models withstand even the most advanced adversarial tactics.
Never stop strengthing data integrity and provenance: Never assume all data is trustworthy. Validate its origins, quality and integrity through rigorous checks and adversarial input testing. By ensuring only clean, reliable data enters the pipeline, SOCs can do their part to maintain the accuracy and credibility of outputs.
Integrate adversarial validation and red-teaming: Don’t wait for attackers to find your blind spots. Continually pressure-test models against known and emerging threats. Use red teams to uncover hidden vulnerabilities, challenge assumptions and drive immediate remediation — ensuring defenses evolve in lockstep with attacker strategies.
Enhance threat intelligence integration: SOC leaders need to support devops teams and help keep models in sync with current risks. SOC leaders need to provide devops teams with a steady stream of updated threat intelligence and simulate real-world attacker tactics using red-teaming.
Increase and keep enforcing supply chain transparency: Identify and neutralize threats before they take root in codebases or pipelines. Regularly audit repositories, dependencies and CI/CD workflows. Treat every component as a potential risk, and use red-teaming to expose hidden gaps — fostering a secure, transparent supply chain.
Employ privacy-preserving techniques and secure collaboration: Leverage techniques like federated learning and homomorphic encryption to let stakeholders contribute without revealing confidential information. This approach broadens AI expertise without increasing exposure.
Implement session management, sandboxing, and zero trust starting with microsegmentation: Lock down access and movement across your network by segmenting sessions, isolating risky operations in sandboxed environments and strictly enforcing zero-trust principles. Under zero trust, no user, device or process is inherently trusted without verification. These measures curb lateral movement, containing threats at their point of origin. They safeguard system integrity, availability and confidentiality. In general, they have proven effective in stopping advanced adversarial AI attacks.
Conclusion
“CISO and CIO alignment will be critical in 2025,” Grazioli told VentureBeat. “Executives need to consolidate resources — budgets, personnel, data and technology — to enhance an organization’s security posture. A lack of data accessibility and visibility undermines AI investments. To address this, data silos between departments such as the CIO and CISO must be eliminated.”
“In the coming year, we will need to view AI as an employee rather than a tool,” Grazioli noted. “For instance, prompt engineers must now anticipate the types of questions that would typically be asked of AI, highlighting how ingrained AI has become in everyday business activities. To ensure accuracy, AI will need to be trained and evaluated just like any other employee.”
Source link